Machine Learning in Business Development: Transforming Strategies with Data-Driven Insights
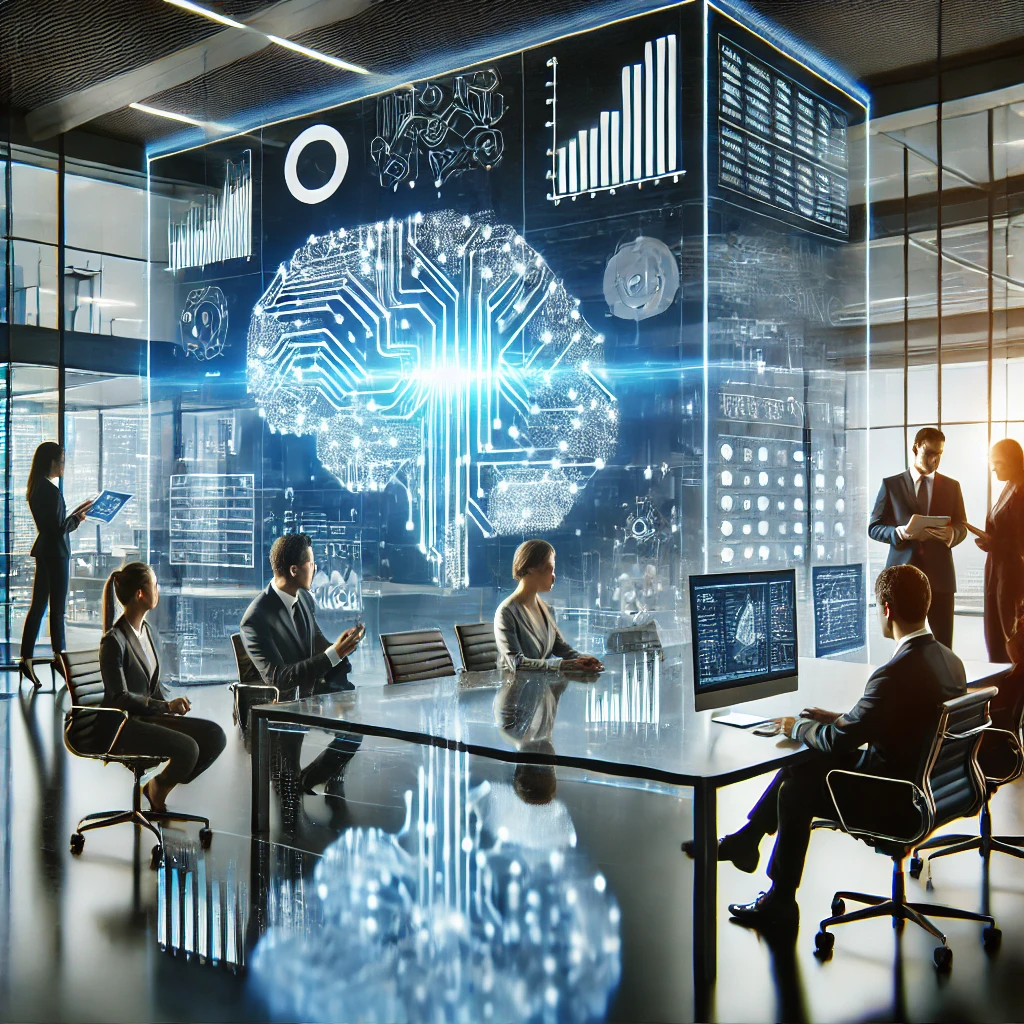
Machine learning (ML) has become a crucial asset for businesses seeking to enhance their operations, make data-driven decisions, and gain a competitive edge. Leveraging ML, companies can analyze massive amounts of data to extract actionable insights, predict trends, and optimize processes. Here’s a look at how machine learning contributes to various aspects of business development:
1. Customer Insights and Personalization
- Behavioral Analysis: ML helps analyze customer behavior to identify patterns, preferences, and needs, enabling companies to create targeted marketing strategies.
- Personalized Recommendations: E-commerce and content platforms use ML to provide personalized product or content recommendations, enhancing user experience and boosting engagement.
- Customer Segmentation: ML algorithms group customers based on demographics, purchase history, and preferences, allowing businesses to tailor their services effectively.
2. Enhanced Marketing and Sales Forecasting
- Predictive Analytics: ML models predict future sales trends and customer demand, enabling companies to plan inventory and production cycles more accurately.
- Customer Lifetime Value (CLV) Prediction: By predicting a customer’s future interactions with a brand, companies can focus on retaining high-value customers.
- Lead Scoring: Machine learning helps prioritize leads by predicting their likelihood of conversion, improving the efficiency of sales teams.
3. Operational Efficiency and Automation
- Process Automation: Machine learning-powered automation improves operational efficiency, reducing human error and freeing up resources for more strategic tasks.
- Supply Chain Optimization: ML algorithms predict demand and supply fluctuations, optimize inventory levels, and improve logistics planning, reducing costs and delays.
- Quality Control: In manufacturing, ML models identify defects or irregularities in real-time, ensuring quality standards and reducing waste.
4. Customer Support and Chatbots
- Automated Customer Service: AI-powered chatbots handle common customer inquiries, reducing wait times and freeing up human agents for more complex issues.
- Sentiment Analysis: ML analyzes customer feedback and reviews to gauge sentiment, helping companies improve customer service and product quality.
- 24/7 Support: Chatbots and virtual assistants provide continuous support, enhancing customer satisfaction and brand loyalty.
5. Fraud Detection and Security
- Fraud Prevention: Machine learning models identify and flag unusual patterns that may indicate fraudulent activities in real-time, protecting businesses and customers.
- Cybersecurity Threat Detection: ML helps detect and respond to cybersecurity threats by recognizing patterns in network activity, preventing data breaches and protecting sensitive information.
- Risk Management: ML enables financial institutions to assess credit risks, helping reduce losses and make informed lending decisions.
6. Product Development and Innovation
- Rapid Prototyping: Machine learning accelerates the product development cycle by enabling rapid testing and iteration of designs based on data and feedback.
- Feature Optimization: ML helps identify the most valuable features for customers, allowing companies to refine and improve products.
- Innovation through Data Analysis: By analyzing market trends, ML provides insights into consumer demands and emerging technologies, guiding product development.
7. Data-Driven Decision Making
- Real-Time Analytics: ML enables real-time data analysis, empowering businesses to make agile, data-driven decisions that adapt to market changes.
- Business Intelligence Enhancement: Integrating ML with BI tools enhances data analysis, making insights easier to understand and act on.
- Reduced Human Bias: ML-driven decision-making reduces human biases, leading to more objective and accurate business strategies.
8. Human Resources and Talent Management
- Recruitment Automation: Machine learning can help in screening resumes, predicting candidate success, and improving the overall hiring process.
- Employee Retention: ML algorithms analyze patterns to predict employee turnover, helping HR departments proactively address issues and retain talent.
- Performance Evaluation: By analyzing employee productivity and performance data, machine learning supports fairer and more objective evaluations.
9. Competitive Analysis and Market Research
- Competitor Tracking: ML tools monitor competitors’ activities, such as pricing changes, new product launches, or customer sentiment, helping businesses stay competitive.
- Trend Prediction: Machine learning models analyze market data to identify trends, enabling companies to capitalize on emerging opportunities.
- Customer Feedback Analysis: ML can rapidly process large volumes of customer feedback, providing insights into customer preferences and competitive advantages.
Responses