The Power Of Bi And Big Data In Modern Business Analysis
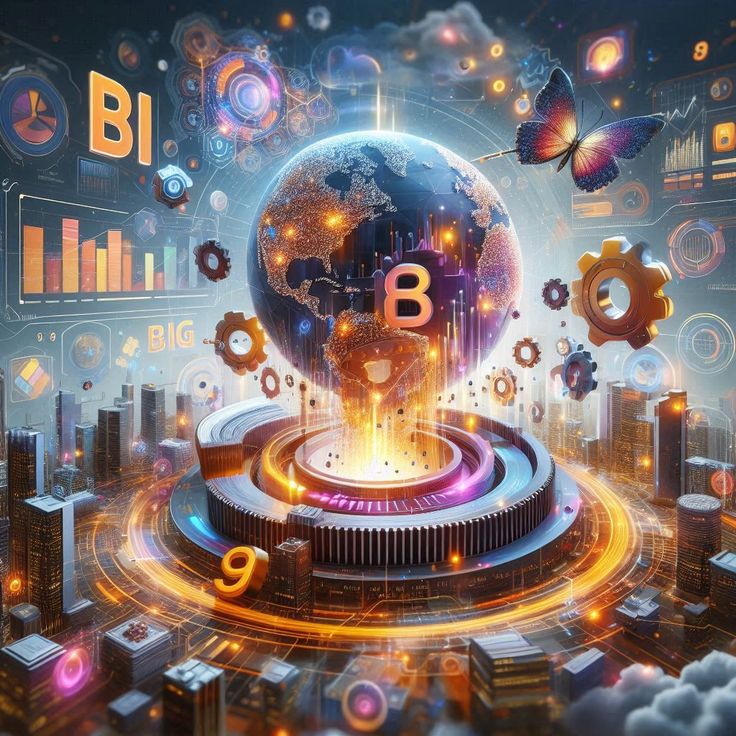
Unlocking the Power of BI and Big Data: A Comprehensive Guide
Welcome to the exciting world of Bi and Big Data! In this article, we will dive deep into the concepts and applications of Bi (Business Intelligence) and Big Data analysis. So, buckle up and get ready to embark on a fascinating journey!
What Are BI and Big Data?
Bi and Big Data are two buzzwords that have been making waves in the business and technology industry in recent years. They have revolutionized the way companies collect, analyze, and interpret data to make informed business decisions. But what exactly do Bi and Big Data mean?
Business Intelligence (BI)
Bi, or Business Intelligence, refers to the process of collecting, analyzing, and presenting data in a way that helps organizations make strategic business decisions. It involves gathering data from various sources, transforming it into meaningful insights, and using these insights to drive business growth and success.
Big Data
Big Data refers to vast and complex datasets that traditional data processing methods cannot handle effectively. This data encompasses structured, semi-structured, and unstructured formats from diverse sources such as social media, sensors, and machines.
Importance of BI and Big Data in Today’s Business Landscape
Now that we have a better understanding of Bi and Big Data, let’s explore why they are so important in today’s rapidly changing business landscape. In the digital age, organizations are generating massive amounts of data every second. This data holds a wealth of insights that can help businesses gain a competitive edge, identify new market opportunities, and improve operational efficiency.
Extracting Valuable Insights
Bi and Big Data analytics enable organizations to extract valuable information from this vast amount of data and turn it into actionable insights. By analyzing patterns, trends, and correlations, businesses can uncover hidden opportunities and make data-driven decisions that drive growth and success.
Techniques and Tools for BI and Big Data Analysis
To harness the power of Bi and Big Data, enterprises need to leverage a wide range of techniques and tools. These include data integration, data warehousing, data mining, data visualization, predictive analytics, and more. These tools help organizations store, process, analyze, and visualize large volumes of data in a structured and meaningful way.
However, integrating Bi and Big Data comes with its own set of challenges. Organizations need to address issues such as data privacy and security, data quality and accuracy, and scalability. They also need to invest in the right infrastructure and talent to effectively manage and analyze Big Data.
Understanding Bi and Big Data Concepts
Alright, let’s dive deeper into the world of Bi and Big Data! Now that we have a basic understanding of what Bi and Big Data are, let’s explore these concepts further.
1. Business Intelligence (Bi)
Business Intelligence, or Bi, refers to the process of analyzing data to gain insights and make informed business decisions. It involves the collection, integration, and analysis of various data sources to provide valuable insights and reports. Bi helps organizations understand their market, customers, operations, and competition better.
With Bi, businesses can track key performance indicators (KPIs) and monitor their performance over time. It allows them to identify trends, patterns, and anomalies in the data, which can help in making data-driven decisions.
2. Big Data
Big Data, on the other hand, refers to large and complex datasets that cannot be easily managed, processed, or analyzed using traditional data processing tools and techniques. It is characterized by the 3Vs: Volume (large amounts of data), Velocity (high speed at which data is generated and processed), and Variety (data from various sources and formats).
Big Data can come from different sources such as social media, sensors, transactional systems, and more. It requires advanced technologies and approaches to store, manage, process, and analyze the data effectively. The insights derived from Big Data can help organizations gain a competitive advantage and make better decisions.
3. Bi vs. Big Data
While Bi focuses on analyzing structured data from internal systems like databases and spreadsheets, Big Data deals with unstructured and semi-structured data from various sources.Â
4. Data Warehousing
Data warehousing plays a crucial role in Bi and Big Data analytics. It is the process of collecting and storing data from various sources into a centralized repository known as a data warehouse. Data warehouses are designed to support Bi and Big Data analytics by providing a single source of truth for the organization’s data.
Data warehousing involves various stages such as data extraction, transformation, and loading (ETL). ETL processes ensure that the data in the data warehouse is clean, consistent, and standardized. This allows organizations to analyze the data effectively and make accurate decisions based on reliable information.
Now that we have a good understanding of the concepts of Bi and Big Data, let’s move on to the next section to learn about the role of Bi in Big Data analytics.
The Role of Bi in Big Data Analytics
Now that we have a basic understanding of Bi and Big Data concepts, let’s explore the role of Bi in Big Data analytics. Bi, or Business Intelligence, refers to the use of data analysis techniques and tools to help businesses make better-informed decisions. On the other hand, Big Data refers to the massive volumes of data that organizations collect from various sources.
By analyzing the Big Data, Bi helps businesses identify patterns, trends, and correlations to make strategic decisions.
Here are some ways in which Bi contributes to Big Data analytics:
1. Data Integration
Bi tools provide the capabilities to integrate and combine data from multiple sources, including structured and unstructured data. This enables organizations to analyze data from various systems and databases, providing a holistic view of their operations. Bi tools can integrate data from internal enterprise systems, external sources, social media, and sensor data, among others.
2. Data Visualization
One of the key aspects of Bi is data visualization, which involves presenting data in a visually appealing and intuitive manner. Big Data analytics generates massive amounts of data, and Bi tools can help businesses make sense of this data by displaying it in the form of charts, graphs, and interactive dashboards. This allows decision-makers to quickly understand the insights and trends hidden within the data.
3. Data Exploration and Analysis
Bi tools provide powerful data exploration and analysis capabilities that enable users to slice and dice the data to gain deeper insights. Through interactive visualizations and drill-down capabilities, users can explore the data from different angles and dimensions, uncovering valuable insights that can drive business decisions. Additionally, Bi tools offer advanced analytics functionalities, such as predictive modeling and data mining, which further enhance the insights derived from Big Data.
4. Real-time Analytics
Bi tools enable real-time analytics by processing and analyzing Big Data in real-time or near-real-time. This allows organizations to monitor their operations, detect anomalies, and respond to events as they happen. Real-time analytics is particularly valuable in industries such as finance, retail, and logistics, where timely decisions can have a significant impact on business outcomes.
5. Performance Management
Bi tools help organizations measure, monitor, and manage their performance based on Big Data insights. By setting key performance indicators (KPIs) and tracking them in real-time, businesses can identify areas for improvement and take corrective actions. Bi tools also facilitate the creation and distribution of performance reports and dashboards, enabling stakeholders at all levels of the organization to stay informed and aligned with the strategic objectives.
Techniques and Tools for Bi and Big Data Analysis
When it comes to analyzing big data, businesses need powerful tools and techniques that can handle the enormous volume, velocity, and variety of data. Fortunately, there are several technologies and methodologies available to help organizations make sense of their big data.
Here are some of the most commonly used techniques and tools in the field of business intelligence and big data analysis:
1. Data Warehousing:
Data warehousing is a technique that involves collecting and storing large amounts of data from various sources into a single, centralized repository. This allows businesses to easily access and analyze their data, making it an essential tool for big data analytics. Data warehousing also enables organizations to integrate data from different systems, such as customer relationship management (CRM) and enterprise resource planning (ERP), providing a comprehensive view of their operations.
2. Data Mining:
Data mining is the process of discovering patterns and insights from large datasets. It involves using statistical techniques and algorithms to identify relationships and trends in the data. Data mining can be used to uncover hidden patterns and correlations that may not be immediately apparent, helping organizations make informed decisions and gain a competitive advantage.
3. Machine Learning:
Machine learning is a subset of artificial intelligence that uses algorithms to analyze and interpret data. It involves training computer systems to automatically learn from and improve upon their performance without being explicitly programmed. Machine learning can be used to predict customer behavior, optimize operations, and make data-driven decisions. It is particularly useful for big data analysis due to its ability to handle large datasets and complex patterns.
4. Hadoop:
Hadoop is an open-source software framework used for processing and storing large volumes of data. It allows businesses to distribute data and processing across multiple computers, making it possible to analyze big data in a distributed manner. Hadoop is designed to be highly scalable and fault-tolerant, ensuring that organizations can handle massive amounts of data without sacrificing performance or reliability.
5. Apache Spark:
Apache Spark is another open-source framework that provides a fast and general-purpose engine for big data processing. It supports real-time data streaming, machine learning, and graph processing, making it a versatile tool for big data analysis. Spark is known for its in-memory processing capabilities, which allow organizations to run complex analytics on large datasets quickly.
6. Data Visualization:
Data visualization is the graphical representation of data to help businesses understand and interpret complex information. It involves creating visualizations such as charts, graphs, and maps to present data in a meaningful and intuitive way. Data visualization tools allow organizations to explore their data visually, identify patterns and trends, and communicate insights effectively.
These techniques and tools are essential for businesses looking to harness the power of big data. By leveraging these technologies, organizations can gain valuable insights, make data-driven decisions, and stay ahead of the competition.Â
Challenges and Issues in BI and Big Data Implementation
Data Quality and Reliability
One of the major challenges in BI and big data integration is ensuring the quality and reliability of the data. Big data is often characterized by its volume, velocity, and variety, which can make it difficult to validate and trust the information. Inaccurate or incomplete data can lead to faulty insights and misguided decision-making. To address this challenge, organizations need to establish robust data governance processes, implement data cleansing techniques, and invest in data quality assurance tools.
Data Security and Privacy
Another critical concern in the integration of BI and big data is ensuring data security and privacy. With increased data collection and analysis comes the risk of unauthorized access, data breaches, and privacy infringements. Organizations need to implement stringent security measures, such as encryption, access controls, and anonymization techniques, to protect sensitive information.Â
Data Integration and Integration Governance
The integration of BI and big data involves consolidating data from various sources, which can be a complex and time-consuming process. Data in different formats and structures need to be harmonized and transformed to ensure compatibility and consistency. Moreover, the integration should be governed to maintain data integrity and reduce duplication or redundancy. Organizations can use data integration tools and establish data integration governance practices to streamline this process.
Scalability and Infrastructure
Big data analytics requires substantial computational power and storage capacity. As organizations accumulate large volumes of data, scalability becomes a significant challenge. The existing IT infrastructure may not be equipped to handle the processing and storage requirements of these extensive datasets. To overcome this challenge, organizations need to invest in scalable and robust infrastructure, such as cloud computing or distributed computing systems, to effectively analyze and manage big data.
Skill Gap and Talent Acquisition
The successful implementation of BI and big data integration relies heavily on the availability of skilled professionals. The field of big data analytics is relatively new and constantly evolving, making it challenging for organizations to find professionals with the desired skillsets. Data scientists, analysts, and developers who understand both BI and big data concepts are in high demand. Organizations need to invest in training programs, collaborate with educational institutions, and use targeted recruitment strategies to bridge the skill gap and attract top talent.
Cost and Return on Investment
Implementing BI and big data integration can be a significant investment for organizations. The costs associated with acquiring hardware, software licenses, hiring skilled professionals, and implementing necessary security measures can add up quickly. It is essential for organizations to carefully evaluate the potential return on investment (ROI) and prioritize investments based on the expected benefits. A well-defined business case and a clear understanding of the value proposition can help organizations make informed decisions while managing costs effectively.
Future Trends and Implications of Bi and Big Data Integration
1. Real-time Decision-making
One of the major trends in the integration of BI and Big Data is the ability to make real-time decisions based on up-to-date information. With the massive amount of data being generated every second, organizations can use BI tools to analyze this data in real time and make informed decisions on the go. This trend will enable businesses to respond quickly to changing market conditions and stay ahead of the competition.
2. Predictive Analytics
Another significant implication of the integration of BI and Big Data is the ability to leverage predictive analytics. By analyzing historical data patterns, organizations can use AI-powered algorithms to predict future outcomes and trends. This will empower businesses to make proactive decisions, anticipate customer needs, and optimize their operations. Predictive analytics will play a crucial role in optimizing marketing campaigns, managing inventory, and improving overall business performance.
3. Enhanced Data Visualization
The integration of BI and Big Data will also lead to enhanced data visualization capabilities. With the help of advanced BI tools, organizations can not only analyze large volumes of data but also present it in a visually appealing and easily understandable manner. Data visualization techniques such as charts, graphs, and heat maps will enable stakeholders to gain insights at a glance, facilitating faster and more accurate decision-making.
4. Continual Learning and Adaptability
As more organizations integrate BI and Big Data, the focus will shift towards continual learning and adaptability. Machine learning algorithms, coupled with Big Data analytics, will enable businesses to continuously analyze data, learn from it, and adapt their strategies accordingly. This iterative approach will result in improved performance, as organizations will be able to identify patterns and trends that were previously unknown, leading to better decision-making and competitive advantage.
5. Increased Data Privacy and Security Measures
The integration of BI and Big Data brings about concerns regarding data privacy and security. In light of recent data breaches and regulations such as the General Data Protection Regulation (GDPR), organizations will need to invest in robust data privacy and security measures. This will ensure that sensitive customer data is protected, and the trust of stakeholders is maintained. Additionally, organizations will need to be transparent about how data is being collected, stored, and used, as privacy concerns are likely to become more prevalent in the future.
Conclusion
In conclusion, the integration of BI and Big Data holds significant future trends and implications for businesses. Real-time decision-making, predictive analytics, enhanced data visualization, continual learning and adaptability, and increased data privacy and security measures are just a few areas that will be impacted. Organizations that successfully harness the power of BI and Big Data integration will gain a competitive edge and be better equipped to navigate the ever-evolving business landscape.
Responses